Biased and hallucinatory AI models can produce unfair results
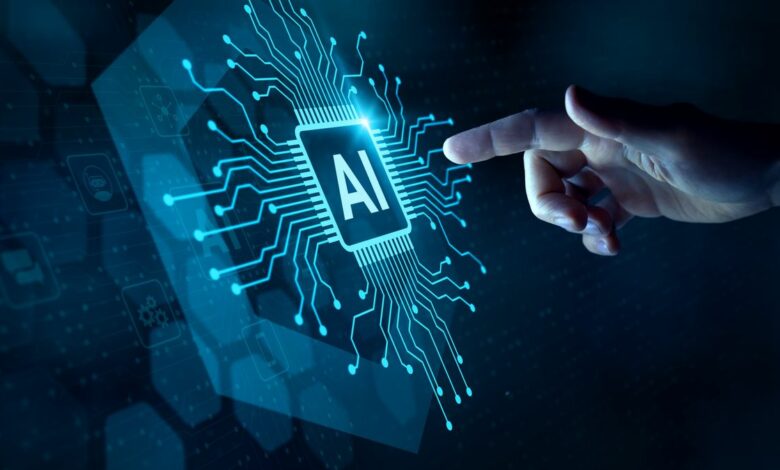
“Code a treasure hunt game for me.” “Cover Psy’s ‘Gangnam Style’ in the style of Adele.” “Create a photorealistic close-up video of two pirate ships battling each other while sailing into a cup of coffee.” Even that last prompt isn’t an exaggeration — today’s best AI tools can create all of this and more in minutes, making AI seem like a genuine form of modern magic.
Of course, we know it’s not magic. A huge amount of work, instruction, and information goes into the models that power GenAI and produce its output. AI systems need to be trained to learn patterns from data: GPT-3, ChatGPT’s base model, was trained on 45TB of Common Crawl data, the equivalent of about 45 million 100-page PDF documents. In the same way that we humans learn from experience, training helps AI models better understand and process information. Only then can they make accurate predictions, perform important tasks, and improve over time.
This means that the quality of the information we feed into our tools is crucial. So how can we ensure that we foster quality data to build practical, successful AI models? Let’s take a look.
COO of Northern Data Group.
The risks of bad data
Good quality data is accurate, relevant, complete, diverse and unbiased. It’s the backbone of effective decision-making, strong operational processes and, in this case, valuable AI outputs. Yet maintaining good quality data is challenging. A survey by a data platform found that 91% of professionals say data quality impacts their organization, yet only 23% cite good data quality as part of their organizational ethos.
Bad data also often contains limited and incomplete information that doesn’t accurately reflect the broader world. The resulting biases can affect how the data is collected, analyzed, and interpreted, leading to unfair or even discriminatory outcomes. When Amazon built an automated hiring tool in 2014 to speed up its hiring process, the software team fed it data on the company’s existing pool of mostly male software engineers. The project was scrapped after just a year when it became apparent that the tool was systematically discriminating against female applicants. Another example is Microsoft’s now-cancelled Tay chatbot, which became infamous for making offensive comments on social media because of the bad data it was trained on.
Returning to AI, messy or biased data can have a similarly catastrophic effect on a model’s productivity. Feeding messy or poor-quality synthetic data into an AI model and expecting it to provide clear, actionable insights is futile; like microwaving a plate of alphabet spaghetti and expecting it to come out spelling “The quick brown fox jumps over the lazy dog.” Data readiness, the state of readiness and quality of data within an organization, is therefore a major hurdle to overcome.
Feeding AI model correctly
Research shows that when it comes to global companies’ AI strategies, only 13% are ranked as leaders in terms of data readiness. Meanwhile, 30% are classified as chasers, 40% as followers, and a disturbingly large 17% as laggards. These figures must change if data is to drive successful AI outcomes worldwide. Ensuring good data readiness requires collecting comprehensive and relevant data from trusted sources, cleaning it to remove errors and inconsistencies, accurately labeling it, and standardizing its formats and scales. Most importantly, we must continuously monitor and update the data to maintain its quality.
To start, companies need to create a centralized data catalog that aggregates data from disparate repositories and silos into one organized location. They then need to classify and curate this data to make it easy to find, use, and mark up contextual business information. Next, engineers need to implement a strong data governance framework that includes regular data quality assessments. Data scientists need to continually detect and correct inconsistencies, errors, and missing values within data sets.
Finally, data lineage tracking involves developing a clear understanding of the data’s origins, processing steps, and access points. This tracking ensures transparency and accountability in the event of a bad outcome. And it becomes especially critical in light of growing concerns about AI privacy.
Ensuring data is fair and secure
Today, personal AI searches are quickly becoming the new confidential Google search. But users would never trust them with private information if they knew it would be shared or sold. According to Cisco research, 60% of consumers are concerned about how organizations use their personal data for AI, while nearly two-thirds (65%) have already lost some trust in organizations as a result of their AI use. So legal concerns aside, we all have an ethical and reputational responsibility to ensure airtight data privacy as we build and leverage AI technology.
Privacy means ensuring that the everyday individuals who interact with AI-powered tools and systems – from healthcare patients to online shoppers – have control over their personal data and can relax knowing that it is being used responsibly. To do this, companies should operate according to a ‘privacy by design’ concept, where their technology only collects data that is strictly necessary, stores it securely, and is transparent about its use.
A good option is to anonymize all collected data. That way, you can reuse it in further AI model training without compromising customer privacy. And once you no longer need this data, you can delete it to eliminate the risk of future breaches. This sounds simple, but it’s an often-overlooked step that can save you significant stress, reputational damage, and even regulatory fines.
Putting data sovereignty first
Compliance with regulatory requirements is of course paramount for any organization. And data residency is a growing focus around the world. In Europe, for example, the GDPR states that EU citizens’ data must reside in the European Economic Area. That means you or your cloud partner will need data centers within the region. If you transfer data anywhere else, you risk breaking the law. Data residency is already a priority for regulators and users alike, and it will only come under increased scrutiny as more regulations roll out around the world.
For businesses, compliance means either purchasing data storage facilities in specific locations outright, or working with a specialized provider that offers data centers in strategic locations. Just ask the World Economic Forum, which says that “the backbone of Sovereign AI is a robust digital infrastructure.” Simply put, data centers with high-performance computing capabilities, operating under policies that ensure generated data is stored and processed locally, are the foundation for the effective, compliant development and deployment of AI technologies worldwide. It’s not quite magic, but the results can be just as impressive.
We provide an overview of the best AI chatbots for businesses.
This article was produced as part of TechRadarPro’s Expert Insights channel, where we showcase the best and brightest minds in the technology sector today. The views expressed here are those of the author and do not necessarily represent those of TechRadarPro or Future plc. If you’re interested in contributing, you can read more here: