Can AI systems truly be human-centric?
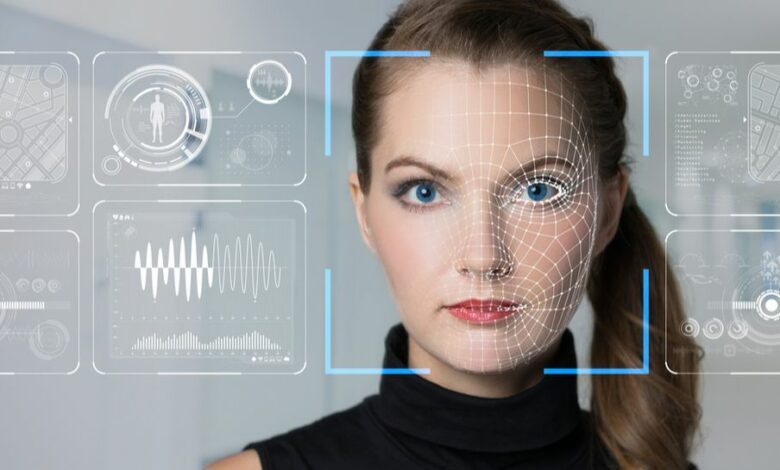
As we enter an era where technology can amplify human potential to unprecedented heights, ensuring trust and accountability within AI is more important than ever. AI innovation, and generative AI in particular, offers tremendous opportunities to address human challenges and problems, but business leaders must proceed with caution.
To address issues like bias and ensure AI delivers real value, the approach to AI tools must be human-centric. Business leaders have a key role to play in how they implement this technology, both for their organizations and for the wider world: AI must serve people, aligned with and embody individual and organizational values, goals and aspirations.
To be responsible, new systems must be human-centered, inclusive, transparent, and accountable. AI systems must be subject to constant oversight and feedback from stakeholders, and must also be designed to work alongside human expertise rather than replace it. AI truly holds the key to improving the way all people interact with technology, but it requires a responsible approach to get the most out of it.
VP Solution Consulting at ServiceNow.
Building AI to solve problems
Generative AI creates value when it solves specific problems for specific people, and this should influence the way AI software is designed. By designing AI software for “personas,” such as people who work in marketing, HR, or finance, people can be placed firmly at the center.
Business leaders need to make sure they understand what each role or persona wants from AI, whether that’s easy-to-use, frictionless experiences or greater agility and productivity. In the case of a developer, that might be code completion or, for an agent, case summarization, which translates more broadly to everything from IT support to customer service to HR.
By taking this human-centric approach and focusing on the real challenges employees and customers face every day, AI-driven solutions can deliver real value across the organization. Continuous feedback from employees and customers helps shape AI solutions that deliver consistent and lasting value, and most importantly, a technology that is fair and open for everyone to use.
Breaking down barriers
Inclusivity and accessibility are essential to improving the way people interact with technology. The ability of multilingual and multimodal AI models to understand everything in multiple languages will be a key way to break down barriers for the people working with AI. Tomorrow’s AI models will be able to understand multiple inputs, such as video and images, meaning technology is more easily accessible regardless of language and location, and enables teams to collaborate across the globe.
AI translation software can also help global teams communicate seamlessly across the enterprise. For agents, this means that customer and employee issues can be resolved faster and in real-time, regardless of the original language in which the issue was raised. For everyone, however, it means that help and understanding can be provided in a more honest way than ever before.
Leveling the playing field
Generative AI interfaces, alongside low-code and no-code applications, can empower business users to be ‘hands-on’ with application development. This not only accelerates digital transformation efforts by enabling business users to build basic digital workflows, but can also increase productivity and job satisfaction, with text-to-code helping anyone create new applications with simple text input.
Generative AI frees up highly skilled developers to focus on more mission-oriented applications. For said developers, AI companions simplify coding and flow construction: this rapid automation can in turn help organizations reduce IT backlogs and drive innovation. For smaller organizations, text-to-code and low-code can be game-changers.
Diversity and Bias
Bias in AI is a real and ongoing problem, affecting everything from credit scores to job applications to the images generated by AI systems. Business leaders must consider the real-world impact of the technology on the people who use and interact with it, and also carefully consider the impact of each AI system on society as a whole. These societal and environmental impacts must be considered at every stage of AI product development.
Both the teams that train, test, and operate such systems, as well as the datasets used in training, must reflect the diversity of society, to ensure that the tools avoid pitfalls such as bias. Business leaders must ensure that AI technology serves the broadest possible range of needs.
It’s important to recognize and be transparent about the tradeoffs that come with adopting AI early on. This, in turn, can spark meaningful conversations with customers about how to navigate challenges, rather than simply wishing them away. To take just one example, it’s impossible to completely eliminate AI bias, but by taking a careful approach to AI training and the teams hired to handle AI, business leaders can recognize and mitigate the problem.
A better future
By putting humans at the center of AI development and taking a transparent, inclusive, and accountable approach, business leaders can build AI systems that truly improve not only their organizations, but also the society around them.
Business leaders must ensure that teams and datasets are diverse, and keep the “human in the loop” with constant feedback from all stakeholders. This collaborative approach is essential for developing safe and accessible AI, with continuous improvement driven by insights and feedback from real-world performance. By centering AI on human needs, human goals, and human values, business leaders can be confident that they can create a better future.
We provide an overview of the best collaboration platforms for teams.
This article was produced as part of TechRadarPro’s Expert Insights channel, where we showcase the best and brightest minds in the technology sector today. The views expressed here are those of the author and do not necessarily represent those of TechRadarPro or Future plc. If you’re interested in contributing, you can read more here: