Liquid AI announces new AI models based on a completely new architecture
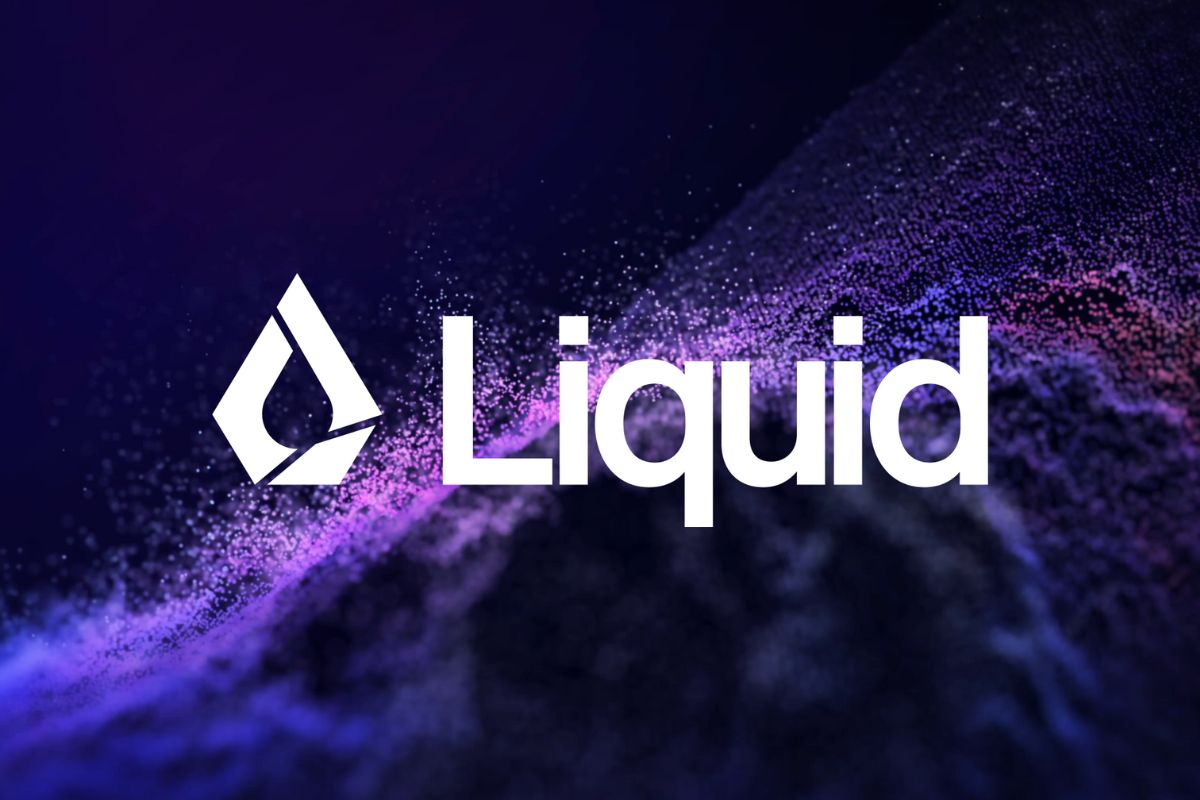
Liquid AI, a Massachusetts-based artificial intelligence (AI) startup, has announced its first generative AI models that do not build on existing transformer architecture. The new architecture, called Liquid Foundation Model (LFM), is a departure from Generative Pre-trained Transformers (GPTs), which are the basis for popular AI models such as OpenAI’s GPT series, Gemini, Copilot, and more. The startup claims that the new AI models are built from first principles and that they outperform large language models (LLMs) of similar size.
Liquid AI’s new Liquid Foundation models
The startup was co-founded in 2023 by researchers at the Computer Science and Artificial Intelligence Laboratory (CSAIL) at the Massachusetts Institute of Technology (MIT) and aimed to build newer architecture for AI models that can perform at a similar level or the Can exceed GPTs. .
These new LFMs are available in three parameter sizes: 1.3B, 3.1B and 40.3B. The latter is a Mixture of Experts (MoE) model, meaning it is made up of several smaller language models and aimed at tackling more complex tasks. The LFMs are now available on the company’s Liquid Playground, Lambda for Chat UI and API, and Perplexity Labs and will soon be added to Cerebras Inference. Furthermore, the AI models are optimized for Nvidia, AMD, Qualcomm, Cerebras and Apple hardware, the company said.
LFMs also differ significantly from GPT technology. The company emphasized that these models are built from first principles. First principles is essentially a problem-solving approach that breaks down a complex technology to its fundamentals and builds from there.
According to the startup, these new AI models are built on so-called computational units. Simply put, this is a redesign of the token system, and the company uses the term Liquid system instead. These contain condensed information with an emphasis on maximizing knowledge capacity and reasoning. The startup claims that this new design helps reduce memory costs during inference and improves performance output for video, audio, text, time series, and signals.
The company further claims that the advantage of the Liquid-based AI models is that the architecture can be automatically optimized for a specific platform based on their requirements and the size of the inference cache.
Although the startup’s clams are large, their performance and efficiency cannot be measured until developers and companies start using them for their AI workflows. The startup did not disclose the source of the datasets or any security measures added to the AI models.