I spoke to Tata Consultancy Services about how new tools can help accelerate AI adoption across a wide range of companies
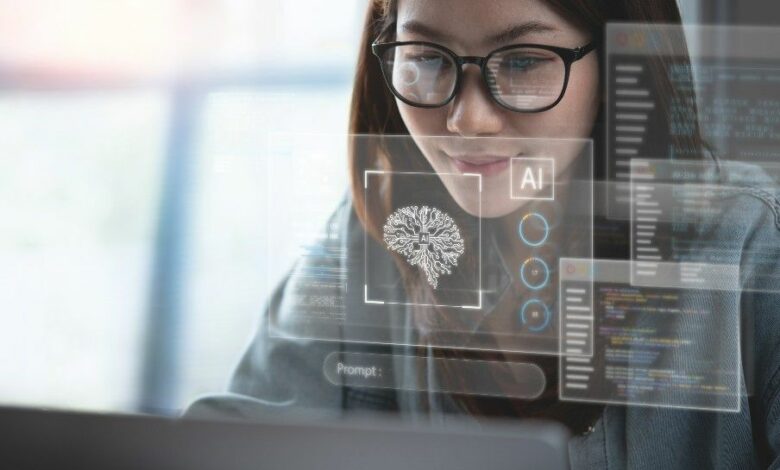
In May 2024, Tata Consultancy Services (TCS) conducted a survey that found that companies wanted to use AI for innovation and revenue growth, but were unsure how to do so.
Now TCS is partnering with Nvidia to help accelerate AI adoption across a range of industries by using the latter’s infrastructure to build customized AI solutions for industries such as manufacturing, automotive, financial and retail, based on large language models.
I spoke with Anupam Singhal, President of Manufacturing at TCS, about how these AI tools work, how they can benefit businesses, and how to mitigate the risks of using AI (if any) in these industries.
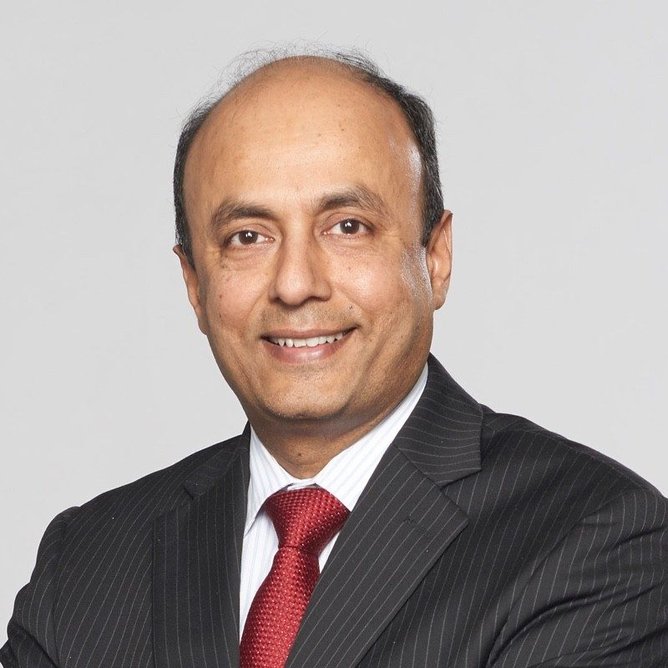
When you think of language models, the topic of manufacturing or the automotive industry doesn’t immediately come to mind. Can you tell me more about the connection between language models and how they can help manufacturing and a range of other industries?
While language models may initially seem more suitable for textual tasks and we often associate B2C use cases with them, they also possess significant potential to revolutionize sectors such as manufacturing and automotive in B2B and B2B2C cases.
We are already seeing this potential come to life through our Future Ready Manufacturing solutions. Here we also transform repair and service cycles and predictive maintenance. We use language models to analyze historical data and identify patterns that can help flag potential equipment failures, reduce downtime and optimize maintenance schedules. Furthermore, using generative AI and SLMs (specialized language models), we can transform the daily activities of repair and service technicians to improve the time required for the repair/service cycle.
Language models also transform supply chain resilience. By analyzing supply chain data, these models can optimize inventory levels, improve logistics and limit supply chain disruptions.
TCS refines LLMs based on industry expertise. What is involved in this process and what safeguards are in place to ensure that the model does not provide sub-optimal suggestions for industry challenges?
Refining LLMs for industry-specific applications is a painstaking process. First, using our industry expertise and customer ecosystem, we curate a high-quality, industry-specific dataset to cover a wide range of scenarios and edge cases. We then use pre-trained and ready-made language models as a basis and refine them based on the industry-specific datasets.
Our engineers then play a crucial role in monitoring the performance of the model, providing feedback and making necessary adjustments. Introducing intuitive adjustments and tacit knowledge increases the accuracy of the model. Once this insight is gathered and entered into the model, we implement strict ethical guidelines to ensure the model generates fair and unbiased results. Appropriate guardrails are defined with TCS’ agent-based monitoring approach to ensure responses are continuously monitored.
Our recently launched TCS Manufacturing AI for industrials suite, built on NVIDIA’s tech stack, is the perfect example of a refined LLM/SLM for this sector.
Although our technology is advanced, we see it as a means to a destination, and not as a destination in itself. The main goal is to transform the daily lives of citizens. Our solutions are designed to help people, not replace them. While AI can perform routine tasks, analyze vast amounts of data, and identify patterns that would otherwise be overlooked, human judgment, creativity, and critical thinking remain essential for making complex decisions and providing strategic direction.
Our goal is to create a synergistic relationship where AI complements human capabilities, allowing us to achieve greater efficiency and innovation. For example, we helped a large building materials company get a quote to market 1.5x faster by using generative AI to provide critical insights on the right configuration and by automating the creation of the draft quote. This enhanced the productivity of those who worked – rather than replacing them.
While it is true that LLMs can produce results that mimic training data and that data generation can be synthetic in some cases, LLMs are also capable of generating new insights and creative solutions to a variety of industry problems. By analyzing massive amounts of data and identifying underlying patterns, these models can uncover hidden opportunities and innovative approaches that may not be apparent to human experts.
For example, TCS is currently building a solution called Mobility AI, part of our TCS Mobility Suite. One use case of this solution is deciphering a parking sign in microsecond intervals and recommending the next best action. These insights enable decisions that humans cannot make at that micro moment in the driver’s journey, highlighting how GenAI can transform this experience in the future of mobility.
However, it is important to note that AI is a tool and its effectiveness depends on how it is used. Human guidance and oversight are critical to ensuring AI is used responsibly and ethically.
Do we risk losing human expertise if we rely more heavily on AI?
Rather than reducing human expertise, AI can actually increase it. By automating routine tasks, AI frees up human workers to focus on higher-value activities that require creativity, problem-solving and empathy. Furthermore, AI can provide valuable insights and support, helping people make more informed decisions. The key is to embrace AI as a tool that empowers people, and not as a threat. By working together, humans and AI can achieve remarkable things.