A business guide to evaluating language models
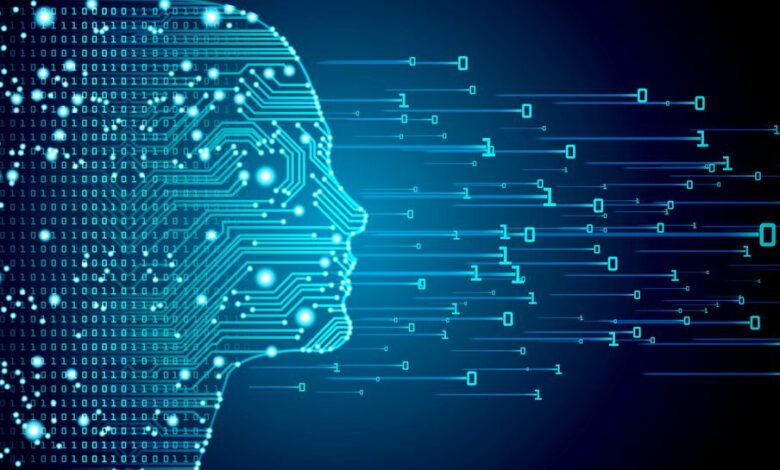
At a time when both the number of artificial intelligence (AI) models and their capabilities are rapidly increasing, enterprises are faced with an increasingly complex challenge: how to effectively evaluate and select the right large language models (LLMs) for their needs.
With the recent release of Meta’s Llama 3.2 and the proliferation of models like Google’s Gemma and Microsoft’s Phi, the landscape has become more diverse (and complicated) than ever before. As organizations attempt to leverage these tools, they must navigate a maze of considerations to find the solutions that best fit their unique requirements.
CTO and co-founder of Iris.ai.
Beyond traditional statistics
Publicly available metrics and rankings often don’t reflect a model’s effectiveness in real-world applications, especially for companies looking to take advantage of deep knowledge locked in their repositories of unstructured data. Traditional evaluation metrics, while scientifically rigorous, can be misleading or irrelevant for business use.
Take Perplexity, a general metric that measures how well a model predicts sample text. Despite its widespread use in academic settings, Perplexity often correlates poorly with actual usability in enterprise scenarios, where its real value lies in a model’s ability to understand, contextualize, and surface actionable insights from complex, domain-specific content.
Companies need models that can navigate the jargon, understand the nuanced relationships between concepts, and extract meaningful patterns from their unique data landscape – capabilities that conventional metrics cannot capture. A model can achieve excellent Perplexity scores but fail to generate practical, business-appropriate responses.
Similarly, BLEU (Bilingual Evaluation Understudy) scores, originally developed for machine translation, are sometimes used to compare the output of language models with reference texts. However, in business contexts where creativity and problem solving are valued, strict adherence to reference texts can be counterproductive. A customer service chatbot that can only respond with pre-approved scripts (which would score well on BLEU) could perform poorly in real customer interactions where flexibility and understanding of context are crucial.
The data quality dilemma
Another challenge in model evaluation arises from training data sources. Most open source models are trained heavily on synthetic data, often generated by advanced models such as GPT-4. While this approach allows for rapid development and iteration, it poses several potential problems. Synthetic data may not fully capture the complexity of real-world scenarios, and its generic nature often does not suit specialized business needs.
Furthermore, when models are evaluated using synthetic data, especially data generated by other language models, there is a risk of creating a self-reinforcing feedback loop that can mask significant limitations. Models trained on synthetic data can learn to replicate artifacts and patterns specific to the generating model, rather than developing a true understanding of the underlying concepts. This creates a particularly challenging situation where evaluation metrics can show strong performance simply because the model has learned to mimic the stylistic quirks and biases of the synthetic data generator rather than demonstrating real capabilities. When training and evaluation rely on synthetic data, these biases can be amplified and become more difficult to detect.
Many business cases require models to be tuned to both industry and domain-specific data to achieve optimal performance. This offers several benefits, including improved performance on specialized tasks and better alignment with business-specific requirements. However, refining is not without challenges. The process requires high-quality, domain-specific data and can be both labor-intensive and technically challenging.
Understanding context sensitivity
Different language models exhibit different levels of performance for different types of tasks, and these differences significantly impact their applicability in different business scenarios. A critical factor in evaluating context sensitivity is understanding how models perform on synthetic versus real data. Models that show strong performance in controlled, synthetic environments may struggle when confronted with the messier, more ambiguous nature of actual business communications. This disparity becomes especially apparent in specialized domains where synthetic training data may not fully capture the complexity and nuance of professional interactions.
Llama models have gained recognition for their strong context preservation and excel at tasks that require coherent, elaborate reasoning. This makes them particularly effective for applications that need consistent context during long interactions, such as complex customer support scenarios or detailed technical discussions.
In contrast, Gemma models, while reliable for many general-purpose applications, may struggle with deep knowledge tasks that require specialized expertise. This limitation can be particularly problematic for companies in areas such as legal, medical or technical domains where deep, nuanced understanding is essential. Phi models present another consideration, as they can sometimes deviate from given instructions. While this characteristic makes them excellent candidates for creative tasks, it requires careful consideration for applications where strict adherence to guidelines is essential, such as in regulated industries or safety-critical applications.
Developing a comprehensive evaluation framework
Given these challenges, companies must develop evaluation frameworks that go beyond simple performance measures. Task-specific performance should be assessed against scenarios directly relevant to the needs of the business. Operational considerations, including technical requirements, infrastructure needs and scalability, play a crucial role. Furthermore, compliance and risk management should not be overlooked, especially in regulated industries where compliance with specific guidelines is mandatory.
Companies should also consider implementing continuous monitoring to detect when model performance deviates from expected standards in production environments. This is often more valuable than initial benchmark scores. Creating tests that reflect actual business scenarios and user interactions, rather than relying solely on standardized academic data sets, can provide more meaningful insights into a model’s potential value.
As AI tools continue to iterate and proliferate, business strategies regarding their valuation and adoption must become increasingly nuanced. While no single approach to model evaluation will meet all needs, understanding the limitations of current metrics, the importance of data quality, and the varying context sensitivity of different models can help organizations select the most appropriate solutions for them. When designing evaluation frameworks, organizations should consider the data sources used for testing. Relying too heavily on synthetic data for evaluation can create a false sense of model capability. Best practices include maintaining a diverse test set that combines both synthetic and real-world samples, with special attention to identifying and checking for any artificial patterns or biases that may be present in synthetic data.
Successful model evaluation lies in recognizing that publicly available benchmarks and metrics are just the beginning. Real-world testing, domain-specific evaluation and a clear understanding of the business requirements are essential for any effective model selection process. By taking a thoughtful, systematic approach to evaluation, companies can navigate AI choices and identify the models that best meet their needs.
We list the best Large Language Models (LLMs) for coding.
This article was produced as part of TechRadarPro’s Expert Insights channel, where we profile the best and brightest minds in today’s technology industry. The views expressed here are those of the author and are not necessarily those of TechRadarPro or Future plc. If you are interested in contributing, you can read more here: