Determining the Right LLM for Your Organization
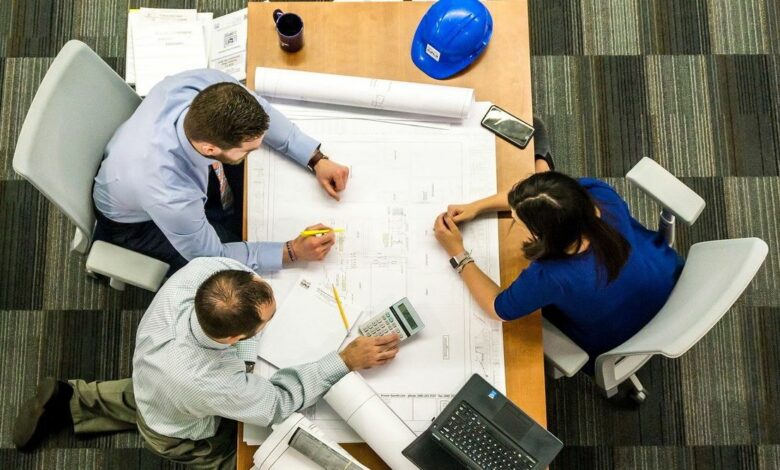
Today’s business leaders recognize that a particular application of generative AI has great potential to drive better business performance, though they may still be investigating how and what the ultimate ROI will be. As companies transform their generative AI prototypes into scaled solutions, they must consider factors such as the technology’s cost, accuracy, and latency to determine its long-term value.
The growing landscape of large language models (LLMs), combined with the fear of making the wrong decision, leaves some companies in a difficult position. LLMs come in all shapes and sizes and can serve different purposes, and the truth is that no single LLM will solve every problem. So how can a company determine which one is right for them?
Here we discuss how to make the best choice so your business can confidently deploy generative AI.
Lead design and strategy at New Relic.
Choose your level of LLM sophistication: the earlier the better
Some companies are conservative in taking on an LLM, launching pilot projects, and waiting for the next generation to see how that might change their application of generative AI. Their reluctance to commit may be justified, as diving in too early and not testing properly can mean big losses. But generative AI is a rapidly evolving technology, with new fundamental models introduced seemingly every week, so being too conservative and waiting for the technology to evolve can mean never really moving forward.
That said, there are three levels of sophistication that companies can consider when it comes to generative AI. The first is a simple wrapper application around GPT, designed to interact with OpenAI’s language models and provide an interface to guide text completion and conversation-based interactions. The next level of sophistication is to use an LLM with retrieval-augmented generation (RAG). RAG allows companies to augment their LLM output with proprietary and/or private data. For example, GPT-4 is a powerful LLM that can understand nuanced language and even reasoning.
However, it is not trained on the data for a specific business, and can lead to potential inaccuracies, inconsistencies, or irrelevances (hallucinations). Businesses can circumvent hallucinations by using implementations like RAG, which allow them to merge insights from a base LLM model with a subset of data unique to their business. (It should be noted that alternative high-context models like Claude 3 could effectively make RAG obsolete. And while many models are still in their infancy, we all know how quickly technology moves, so obsolescence may occur sooner rather than later.)
At the third level of generative AI refinement, a company runs its own models. For example, a company might take an open-source model, refine it with proprietary data, and run the model on its own IT infrastructure instead of third-party offerings like OpenAI. It should be noted that this third-level LLM requires supervision by engineers trained in machine learning.
Apply the right LLM to the right use case
Given the options here and the differences in cost and capabilities, businesses need to determine exactly what they plan to achieve with their LLM. For example, if you are an ecommerce business, your human support team is trained to intervene when a customer is at risk of abandoning their cart and help them decide to complete their purchase. A chat interface will achieve the same result for a tenth of the cost. In this case, it may be worth it for the ecommerce business to invest in running their own LLM with engineers to manage it.
But bigger isn’t always cost-effective — or even necessary. If you’re a banking application, you can’t afford to make transaction errors. That’s why you want more control. By developing your own model or using an open-source one, fine-tuning it, applying heavily designed input and output filters, and hosting it yourself, you get all the control you need. And for those companies that simply want to optimize the quality of their customer’s experience, a high-performing LLM from a third-party vendor would work well.
A note on observability
Regardless of the LLM chosen, it’s important to understand how the model is performing. As tech stacks become increasingly complex, it can be difficult to identify performance issues that may crop up in an LLM. Additionally, due to the uniqueness of the tech stack and the very different LLM interactions, there are entirely new metrics to track, such as time-to-token, hallucinations, bias, and drift. That’s where observability comes in, providing end-to-end visibility across the stack to ensure uptime, reliability, and operational efficiency. In short, adding an LLM without visibility can have a major impact on how a company measures the ROI of its technology.
The generative AI journey is exciting and fast-paced — if not a little daunting. Understanding your business needs and matching them with the right LLM will not only provide short-term benefits, but also lay the foundation for ideal future business outcomes.
We offer the best AI tools.
This article was produced as part of TechRadarPro’s Expert Insights channel, where we showcase the best and brightest minds in the technology sector today. The views expressed here are those of the author and do not necessarily represent those of TechRadarPro or Future plc. If you’re interested in contributing, you can read more here: