How to make your data smart
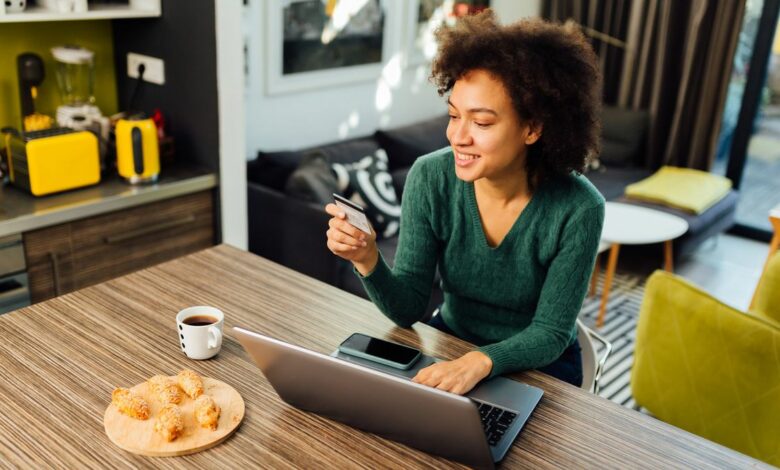
At a recent FinTech North event, I had the opportunity to speak on a panel discussion and delve into one of my favorite topics: the value of smart data.
What is it exactly? Data is not inherently smart or not – but how you use it can be. Only when you build the right processes and pipelines around data does it become a strategic business asset.
Unlock smart data value with cleansing and standardization
The first part of any data process is to make sense of it. Cleaning raw data ensures consistency and accuracy, making it easier to compare multiple sources to gain meaningful insights. Companies then use this data to create a variety of reports, both for internal use and to meet external regulatory requirements.
When the right data quality processes are in place, the reconciliation and reporting process is more effective. Without these processes, teams are overloaded with time-consuming (and avoidable) downstream data work. Companies should strive to be able to standardize data from many different sources into a common language.
At Kani Payments we get data from multiple sources, mostly processors that work with Mastercard or Visa card issuers. We pull their transaction data into our system. Mostly, payment gateways provide data in their own format for use by their customers, who are the ones coming to us.
Head of Data Science at Kani Payments.
We extract data from multiple files that come in different formats and import it into a database, which structures the data. An additional layer of standardization allows us to use all available transaction data for reporting. By making it accessible via user-friendly dashboards, our customers get a standardized overview, so they can consult all their data in one place.
Getting this holistic view should be the main goal of your data strategy, especially if you’re working with multiple payment processors. One processor might give you a CSV file, another an XML file, and yet another a PDF or text file. Internal finance teams tend not to be as tech-savvy — they don’t understand these different file formats, or how to open them and get the information they really want. The right solution does all of this for you.
How Data Standardization Improves Business Efficiency
A robust data governance framework means that finance teams don’t have to spend all day trying to understand their data through manual, time-consuming data analysis tasks. A platform like Kani can import files in seconds, map them into a single schema, and produce actionable analysis. Teams don’t have to wade through thousands of files before their data is actionable, and can instead focus on tasks that add real business value.
With smart processes, our customers can interrogate their data and underlying trends in cardholder behavior. Examples include looking at what cardholders spend, where they spend, and what method they use (chip and pin, contactless, online purchases, etc.). Standardized data offers much more flexibility in reporting on these metrics.
Once the data is easy to digest, you can generate custom reports for tailored business insights. One report we developed looked at supermarket spend, allowing the client to see the effect of lockdowns as people moved from smaller, more frequent shops to a more substantial weekly shop. Creating these custom reports would have been a labour-intensive task using internal resources.
Effective data standardization processes help speed investigations and correct anomalies in reconciliations. With so many different files and data sets involving multiple sources, it is common for some data fields to be missing. Or perhaps one processor generates data columns with a certain name (such as transaction date, currency, and transaction amount), while another uses a random code for the same column. These differences create problems with reconciliations.
If customers wanted to investigate these differences, they would have to dig through each processor’s specification document and dig through every column in each data set to find what they were looking for. Advanced standardization practices solve that by looking for certain transaction elements, such as dates, amounts, or how the transaction was authorized.
Smart data can become even smarter
All of our work in the payments industry is about providing solutions for our clients to get the most value from their data, and we’re excited to see our work here extend into other areas. We’ve started mapping our client data to broader economic data, such as GDP figures or other macroeconomic data, to provide more intelligent insights and benchmark against the broader industry.
Internally, we do some really interesting trend analysis on countries and payment trends. We enable clients to see that on a visual level. If clients want to enter a new market, we can look at their data, analyze regional trends from other data sources and determine what’s best for them.
Looking ahead, we plan to apply different statistical models or machine learning models for more accurate predictions. You might have a set of cardholder spending at a certain point in time, but what will that look like in six months? That’s the next step to extract more actionable information from your data and then use it to drive management decisions.
I often get asked what the future of data science will look like. In one word: busy!
Data science will continue to attract more and more recruitment simply because there is so much potential to learn from it. It will only become more mainstream as people become more aware of techniques such as machine learning, especially with the rise of ChatGPT and other large language models. As more people become interested in these areas, it will help to build more research, development, and interest in potential applications.
We offer the best credit card processing for small businesses.
This article was produced as part of TechRadarPro’s Expert Insights channel, where we showcase the best and brightest minds in the technology sector today. The views expressed here are those of the author and do not necessarily represent those of TechRadarPro or Future plc. If you’re interested in contributing, you can read more here: