Is AI the next step in cloud vendor lock-in?
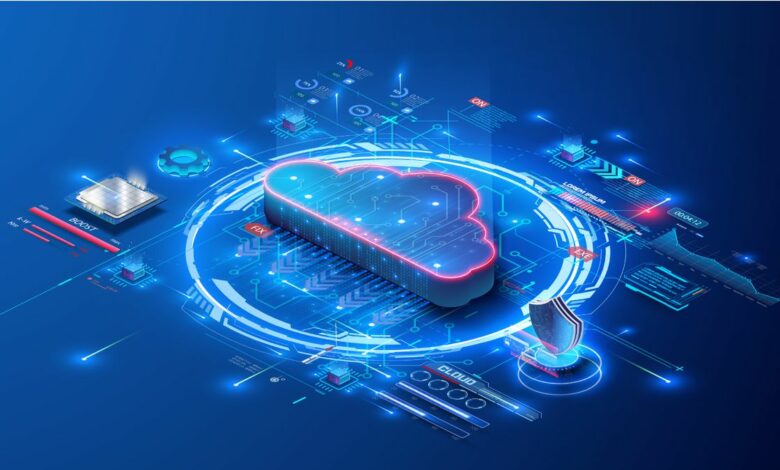
In October 2022, the UK communications regulator Ofcom launched an investigation into the practices of the cloud hyperscalers. Since then, Google, Amazon and Microsoft have been referred to the Competition and Markets Authority (CMA) – the antitrust regulator – putting the issue of cloud vendor lock-in firmly in the spotlight.
There have been multiple iterations of this story, but recently the CMA published the findings of qualitative interviews with hyperscaler customers as the first phase of its research. As many in the industry expected, interoperability was the top concern, with technical barriers making moving data or services between cloud computing services a significant challenge. Egress fees – the charges levied for moving data out of a provider’s cloud – were somewhat surprisingly seen as less of an issue, with many of the organisations interviewed describing them as negligible.
However, this indifference towards exit costs changed when AI was asked.
Regional Vice President Cloud – EMEA & APAC at Cloudera.
A look into the AI-driven future
Over the past two years, AI – particularly generative AI – has dominated the tech landscape. Organizations are now beginning to move from the experimental lab phase of AI implementation to production deployment with some initial use cases.
The hyperscalers have been at the forefront of AI investment. Microsoft invested $13 billion in OpenAI last year, setting the tone for a trend that has now seen the big three hyperscalers invest heavily. It’s quickly becoming a major battleground, with each now able to claim exclusivity on certain AI products.
So naturally, the CMA raised questions about how cloud providers will impact AI in the future. This has been identified as a potential issue, particularly when it comes to egress costs. Currently, the amount of data that organisations move from one cloud to another is relatively small, hence the negligible egress costs. However, if an enterprise had the majority of its data in a hyperscaler’s environment but wanted to use another cloud provider to access its AI tools, the egress costs of moving that volume of data would be astronomical.
Organizations may want to deploy or train AI models on different hyperscalers for certain tasks. This means that organizations need the ability to easily move sets of data from one cloud to another. Here, the constant flow of data back and forth would result in both egress costs and interoperability issues. This means that organizations are faced with the choice of either sticking with their current provider and only using the AI tools they offer, or spending months preparing their data for migration. One client featured in the CMA interim report made this exact point:
“One of the things that’s a concern right now is lock-in. So for our analytics work, we’ve been using AWS, their tooling, their modeling, and the lock-in in terms of AI feels much stronger than with other services. For example, optimizing certain models on certain clouds would make it very difficult, I think, to go anywhere else. But it’s certainly something we’re looking at more. I don’t think we understand what the answer is at this point. But it is a concern of ours, and the lock-in is a big concern because I think it’s pushing us toward a certain way of using AI with certain models.”
Breaking Cloud Barriers
The interim report makes it clear that while there is currently a lock-in to a single cloud vendor, interoperability issues and outbound connectivity costs will only increase as AI becomes more widely adopted.
To be clear, a more flexible cloud market will not result in a switching frenzy where organizations move all of their data regularly. Because even if the CMA takes action to address interoperability issues, moving all of an organization’s data between clouds would still be a monumental task.
More likely, customers will move subsets of data from one cloud to another depending on which AI tool they want to use – essentially adopting a very flexible multi-cloud model. Here, hyperscalers will likely see as much data coming into their environments as going out, with smaller data sets being moved more frequently.
That is why a modern data architecture is essential for organizations that want to use AI effectively in the future.
Whether they’re switching cloud providers entirely or moving a subset of data from one hyperscaler to another to take advantage of AI, a unified data platform can help by providing an abstraction layer that allows them to move data more easily and securely from one cloud to another.
Hyperscalers should not be gatekeepers of AI innovation
Choice and flexibility over which AI products to use are vital, especially as organisations are likely to want to innovate with AI. But progress should not be unnecessarily constrained by a few companies. The ethos of the cloud has always been one of flexibility and bringing people together. This should be reflected in organisations’ freedom of choice over how they use AI. It will then be up to customers to ensure they can move data between clouds as and when they need to.
We provide an overview of the best cloud cost management services.
This article was produced as part of TechRadarPro’s Expert Insights channel, where we showcase the best and brightest minds in the technology sector today. The views expressed here are those of the author and do not necessarily represent those of TechRadarPro or Future plc. If you’re interested in contributing, you can read more here: