Microsoft made a conscious decision to use old technology for its Nvidia GPU rival: the Maia 100 AI accelerator uses HBM2E memory and the mysterious ability to “unlock new capabilities” via a firmware update
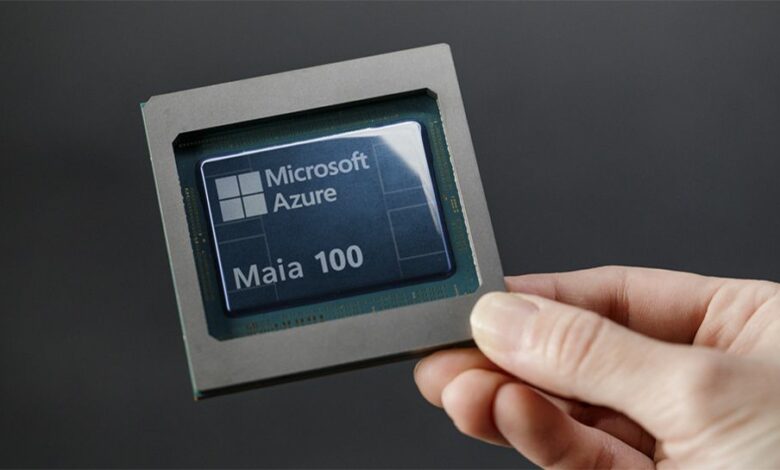
At the recent Hot Chips 2024 symposium, Microsoft revealed details about its first-generation custom AI accelerator, the Maia 100, which is designed for large-scale AI workloads on its Azure platform.
Unlike its rivals, Microsoft has opted for older HBM2E memory technology, integrated with the intriguing ability to “unlock new capabilities” via firmware updates. This decision seems like a strategic move to balance performance and cost-efficiency.
The Maia 100 accelerator is a reticle-size SoC built on TSMC’s N5 process and featuring a COWOS-S interposer. It includes four HBM2E memory chips, delivering 1.8 TBps of bandwidth and 64 GB of capacity, tailored for high-throughput AI workloads. The chip is designed to support up to 700W TDP but is powered by 500W, making it power efficient for its class.
“Not as capable as an Nvidia H100”
Microsoft’s approach with Maia 100 emphasizes a vertically integrated architecture, from custom server boards to specialized racks and a software stack designed to enhance AI capabilities. The architecture includes a fast tensor unit and a custom vector processor, which supports multiple data formats and is optimized for machine learning needs.
Additionally, the Maia 100 supports Ethernet-based connections with an all-gather and scatter-reduced bandwidth of up to 4800 Gbps, utilizing a custom RoCE-like protocol for reliable, secure data transfer.
Patrick Kennedy of ServeTheHome reported on Maia at Hot Chips, noting: “It was really interesting that this is a 500W/700W device with 64GB of HBM2E. You might expect it not to be as capable as an Nvidia H100, given that it has less HBM capacity. At the same time, it uses a fair amount of power. In today’s power-constrained world, it seems like Microsoft should be able to make these a lot cheaper than Nvidia GPUs.”
The Maia SDK simplifies implementation by allowing developers to port their models with minimal code changes, and supports both PyTorch and Triton programming models. This allows developers to optimize workload performance across different hardware backends without sacrificing efficiency.