New technology promises to add terabytes of memory (or SSD storage) to any GPU, but there’s a big catch
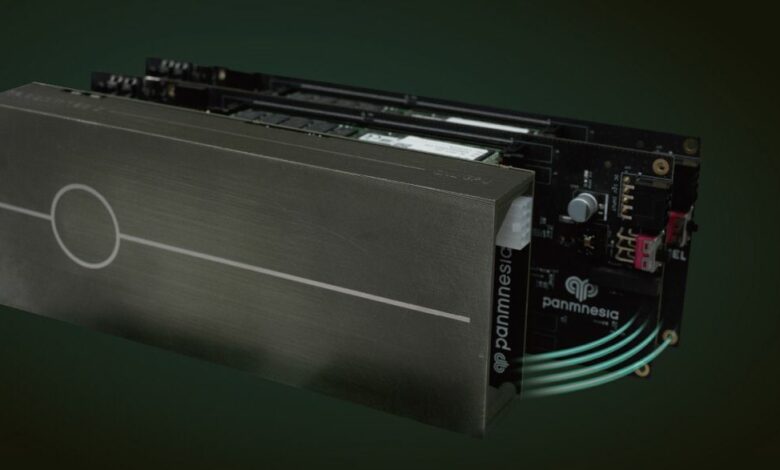
KAIST Startup Panmnesia (the name means “the ability to remember absolutely everything you think, feel, encounter and experience”) claims to have developed a new approach to improving GPU memory.
The company’s breakthrough makes it possible to add terabyte-scale memory using cost-effective storage media such as NAND-based SSDs, while maintaining reasonable performance levels.
There’s a catch, though: the technology is based on the relatively new Compute Express Link (CXL) standard, which is unproven in many common applications and requires specialized hardware integration.
Technical challenges remain
CXL is an open standard interconnect designed to efficiently connect CPUs, GPUs, memory, and other accelerators. It allows these components to share memory coherently, meaning they can access shared memory without having to copy or move data, reducing latency and increasing performance.
Because CXL is not a synchronous protocol like JEDEC’s DDR standard, it can accommodate different types of storage media without the need for exact timing or latency synchronization. Panmnesia says initial tests have shown that the CXL GPU solution can outperform traditional GPU memory expansion methods by more than three times.
For its prototype, Panmnesia connected the CXL Endpoint (which contains terabytes of memory) to its CXL GPU via two MCIO (Multi-Channel I/O) cables. These high-speed cables support PCIe and CXL standards, allowing for efficient communication between GPU and memory.
However, adoption may not be straightforward. GPU cards may require additional PCIe/CXL-compatible slots, and significant technical challenges remain, particularly with the integration of CXL logic and subsystems into current GPUs. Integrating new standards such as CXL into existing hardware requires ensuring compatibility with current architectures and developing new hardware components, such as CXL-compatible slots and controllers, which can be complex and resource-intensive.
While Panmnesia’s new CXL GPU prototype promises potentially unparalleled memory expansion for GPUs, its reliance on the emerging CXL standard and the need for specialized hardware may create barriers to immediate widespread adoption. Despite these obstacles, the benefits are clear, especially for large-scale deep learning models that often exceed the memory capabilities of current GPUs.